Assistant Professor
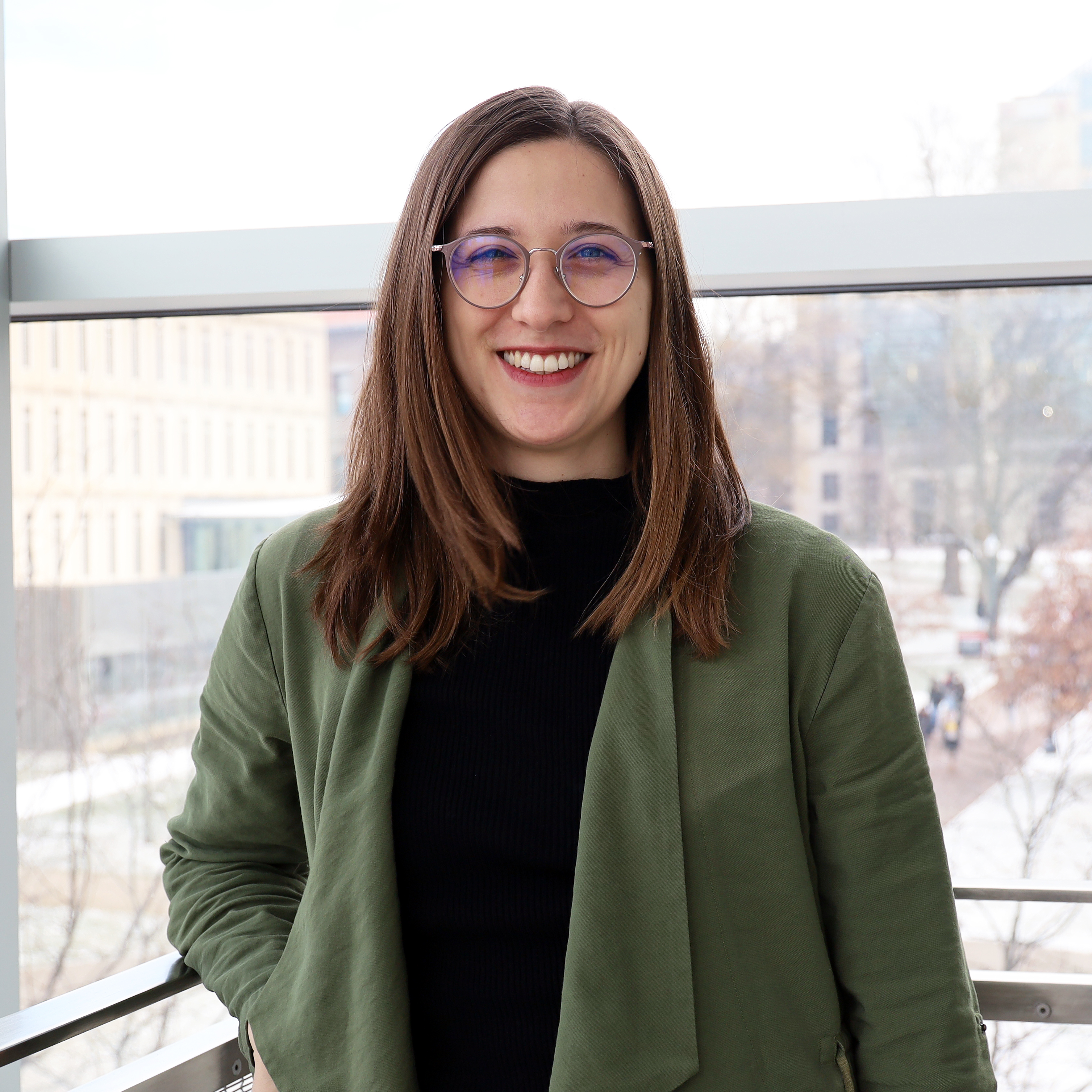
As a Latina and first-generation college student, I personally know the power of transformation that access to information, education and research opportunities can have. Biostatistics allows me to contribute to public health by planning and analyzing studies, in addition to developing statistical methods to handle complex problems.
Contact
210 Cunz Hall
1841 Neil Ave.
Columbus, OH 43210
Email: schumacher.313@osu.edu
Pronouns: she/her/hers
View CV
Website:
Personal website
Dr. Schumacher's primary research interest has been developing robust statistical models that can accommodate specific characteristics frequently found in applications, such as outliers, skewness, time dependence, and censoring. She has experience working with regression and mixed models in the context of time series and longitudinal data, mainly from a frequentist point of view. Most of her work is available as open-source R packages, facilitating the use of these flexible models in applications.
- Censored regression models
- Linear and nonlinear mixed-effects models
- Longitudinal models
- Missing data analysis
- Multiple sclerosis disease
- Robust models
- Skew-elliptical/elliptical distributions
- Variable selection for mixed models
- Ph.D., Statistics, University of Campinas (Brazil), 2021
- M.Sc., Statistics, University of Campinas (Brazil), 2016
- B.Sc., Statistics, State University of Maringa (Brazil), 2014
Schumacher, F. L., Lachos, V. H., Matos, L. A. (2021). Scale mixture of skew‐normal linear mixed models with within‐subject serial dependence. Statistics in Medicine, 40(7), 1790-1810.
Schumacher, F. L., Dey, D. K., Lachos, V. H. (2021). Approximate inferences for nonlinear mixed effects models with scale mixtures of skew-normal distributions. Journal of Statistical Theory and Practice, 15(3), 1-26.
Schumacher, F. L., Ferreira, C. S., Prates, M. O., Lachos, A., Lachos, V. H. (2021). A robust nonlinear mixed-effects model for COVID-19 death data. Statistics and Its Interface, 14, 49-57.
Schumacher, F. L., Lachos, V. H., Vilca-Labra, F. E. (2018). Influence diagnostics for censored regression models with autoregressive errors. Australian & New Zealand Journal of Statistics, 60(2), 209-229.
Schumacher, F. L., Lachos, V. H., Dey, D. K. (2017). Censored regression models with autoregressive errors: A likelihood-based perspective. The Canadian Journal of Statistics, 45, 375-392.