Chair and Professor
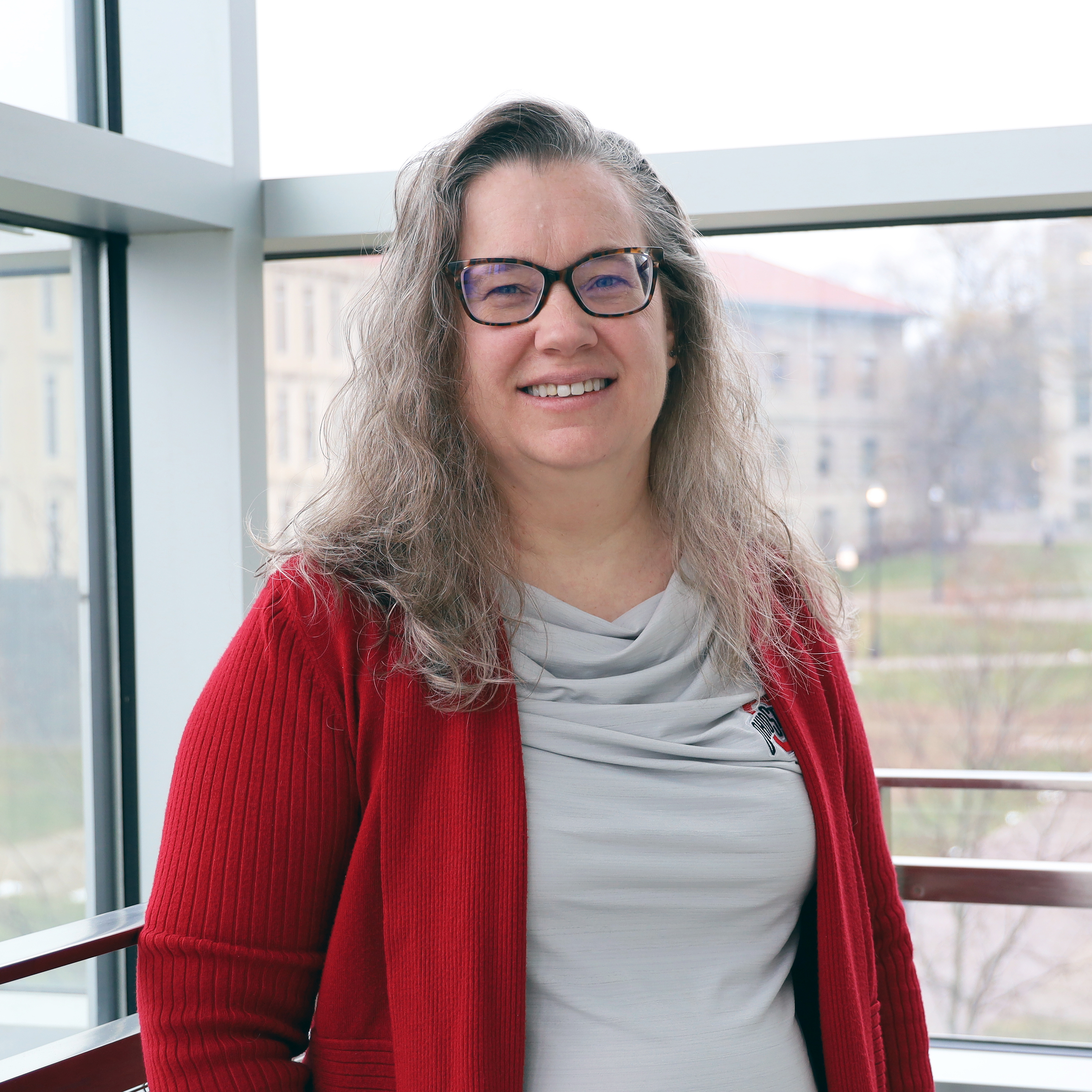
It is essential to conduct research, make evidence-based decisions and derive policies in public health using data from well-designed studies that are appropriately analyzed. Working as a biostatistician at Ohio State allows me to engage in a wide range of applications that are seeking to reduce disease burden and improve public health. Additionally, we are training the next generation of biostatistical researchers to advance methodologies for solving new statistical challenges posed by collecting complex, high-dimensional and high-volume data.
Contact
1841 Neil Ave.
240 Cunz Hall
Columbus, OH 43210
Email: archer.43@osu.edu
Phone: 614-247-6167
View CV
Website:
Github webpage
Dr. Archer’s primary research area has been in the development of statistical methods and computational algorithms for analyzing high-dimensional datasets. Such datasets frequently arise in studies that use high-throughput genomic assays, which yield datasets consisting of a large number of candidate predictors (p, the number of genes or proteins) on a small number of observations (n, the number of samples). Therefore, fitting statistical models for overparameterized problems is an active area of methodological research. Her current research is focused on developing methods and software when the response is either ordinal, discrete, or a time-to-event response where subjects may also experience cure and the genomic data are collected cross-sectionally or longitudinally.
Statistical methods for the analysis of data from high-throughput genomic assays; Discrete and ordinal response modeling; Mixture cure models; Statistical computing; Supervised learning and data mining
ACTIVE PI-level grants:
- R01LM013879 Penalized mixture cure models for identifying genomic features associated with outcome in acute myeloid leukemia
- R21AI172077 Pretransplant comprehensive scores to predict long term graft outcomes
- R01DK109581 Assessment of Donor Quality for Improving Kidney Transplant Outcomes
- statistical methods for high-dimensional datasets
- genomics
- mixture cure models
- ordinal response models
- PhD, College of Public Health, The Ohio State University, 2001
- Master Applied Statistics, Department of Statistics, The Ohio State University, 1993
- BA, Applied Mathematics & Philosophy, Franklin College, 1991
Elected Fellow, American Statistical Association
Selected PubMed Listing (Student names are underlined)
Seffernick AE, Mrózek K, Nicolet D, Stone RM, Eisfeld AK, Byrd JC, Archer KJ. High-dimensional genomic feature selection with the ordered stereotype logit model. Briefings in Bioinformatics, Nov 19;23(6):bbac414, 2022.
Fu H, Nicolet D, Mrózek K, Stone RM, Eisfeld AK, Byrd JC, Archer KJ. Controlled variable selection in Weibull mixture cure models for high-dimensional data. Statistics in Medicine, Sep 30;41(22):4340-4366, 2022.
Archer KJ, Bardhi E, Maluf DG, McDaniels J, Rousselle T, King A, Eason JD, Gallon L, Akalin E, Mueller TF, Mas VR. Pretransplant kidney transcriptome captures intrinsic donor organ quality and predicts 24-month outcomes. American Journal of Transplantation, 22(11):2515-2528, 2022.
Archer KJ, Seffernick AE, Sun S, Zhang Y. ordinalbayes: Fitting Ordinal Bayesian Regression Models to High-Dimensional Data Using . Stats (Basel), Jun;5(2):371-384, 2022.
Zhang Y, Archer KJ. Bayesian penalized cumulative logit model for high-dimensional data with an ordinal response. Statistics in Medicine, Mar 15;40(6):1453-1481, 2021.
Zhang Y, Archer KJ. Bayesian variable selection for high-dimensional data with an ordinal response: identifying genes associated with prognostic risk group in acute myeloid leukemia. BMC Bioinformatics, Nov 2;22(1):539, 2021.
Fu H, Archer KJ. High-dimensional variable selection for ordinal outcomes with error control. Briefings in Bioinformatics, Jan 18;22(1):334-345, 2021.
Lehman RR, Archer KJ. Penalized negative binomial models for modeling an overdispersed count outcome with a high-dimensional predictor space: Application predicting micronuclei frequency. PLoS One,14(1):e0209923, 2019.
Siangphoe U, Archer KJ, Mukhopadhyay ND. Classical and Bayesian random-effects meta-analysis models with sample quality weights in gene expression studies. BMC Bioinformatics, 20:18, 2019.
Siangphoe U, Archer KJ. Estimation of random effects and identifying heterogeneous genes in meta-analysis of gene expression studies. Briefings in Bioinformatics, 18(4):602-618, 2017.
Gentry AE, Jackson-Cook C, Lyon D, Archer KJ. Penalized Ordinal Regression Methods for Predicting Stage of Cancer in High-Dimensional Covariate Spaces. Cancer Informatics, 14(Suppl 2):201-8, 2015.
Makowski M, Archer KJ. Generalized monotone incremental forward stagewise method for modeling count data: Application predicting micronuclei frequency. Cancer Informatics, 14(Suppl 2):97-105, 2015.
Ferber K, Archer KJ. Modeling discrete survival time using genomic feature data. Cancer Informatics, 14(Suppl 2):37-43, 2015.
Hou J, Archer KJ. Regularization method for predicting an ordinal response using longitudinal high-dimensional genomic data. Statistical Applications in Genetics and Molecular Biology, 14(1):93-111, 2015.
Archer KJ, Hou J, Zhou Q, Ferber K, Layne JG, Gentry AE. ordinalgmifs: An R package for ordinal regression in high-dimensional data settings. Cancer Informatics, 13:187-95, 2014.
Archer KJ, Williams AAA. L1 penalized continuation ratio models for ordinal response prediction using high-dimensional datasets. Statistics in Medicine, 31(14):1464-74, 2012.
Asomaning N, Archer KJ. High-throughput DNA methylation datasets for evaluating false discovery rate methodologies. Computational Statistics and Data Analysis, 56(6):1748-1756, 2012.
Archer KJ, Zhao Z, Guennel T, Maluf DG, Fisher RA, Mas VR. Identifying genes progressively silenced in preneoplastic and neoplastic liver tissues. International Journal of Computational Biology and Drug Design, 3(1), 52-67, 2010.
Archer KJ, Reese SE. Detection Call Algorithms for High-throughput Gene Expression Microarray Data. Briefings in Bioinformatics, 11(2):244-52, 2010.
Archer KJ, Mas VR. Ordinal response prediction using bootstrap aggregation, with application to a high-throughput methylation dataset. Statistics in Medicine, Dec 20;28(29):3597-610, 2009.
Archer KJ and Kimes RV. Empirical characterization of random forest variable importance estimates. Computational Statistics and Data Analysis, 52(4): 2249-2260, 2008.
Archer KJ, Dumur CI, Joel SE, Ramakrishnan V. Assessing quality of hybridized RNA in Affymetrix GeneChip experiments using mixed effects models. Biostatistics, 7(2):198-212, 2006.