Erin Hartman, PhD, Berkeley
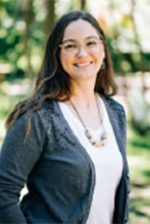
Erin Hartman, PhD
Assistant Professor
University of California, Berkley Department of Political Science
https://erinhartman.com/
Survey weighting allows researchers to account for bias in survey samples, due to unit nonresponse or convenience sampling, using measured demographic covariates. Unfortunately, in practice, it is impossible to know whether the estimated survey weights are sufficient to alleviate concerns about bias due to unobserved confounders or incorrect functional forms used in weighting. In the following paper, we propose two sensitivity analyses for the exclusion of important covariates: (1) a sensitivity analysis for partially observed confounders (i.e., variables measured across the survey sample, but not the target population), and (2) a sensitivity analysis for fully unobserved confounders (i.e., variables not measured in either the survey or the target population). We provide graphical and numerical summaries of the potential bias that arises from such confounders, and introduce a benchmarking approach that allows researchers to quantitatively reason about the sensitivity of their results. We demonstrate our proposed sensitivity analyses using state-level 2020 U.S. Presidential Election polls.
Zoom link: https://osu.zoom.us/j/94300135379?pwd=aElUeitFRDd6WTZhRHlicyttMkMwdz09