Series Link
When
-
Where
160 Cunz Hall and Zoom
Speaker(s)
Pallavi Ray
Research Scientist
Eli Lilly and Company, Indianapolis, Indiana
https://www.linkedin.com/in/pallavi-ray-467449224
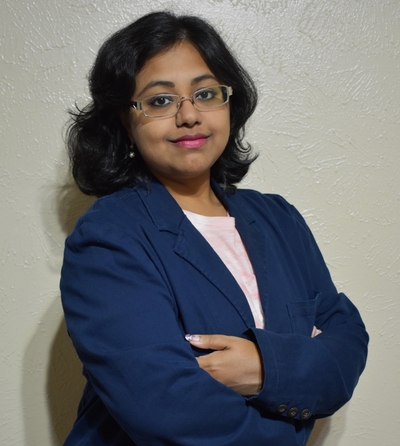
In diverse application areas, it is often of interest to estimate a function nonparametrically subject only to certain restrictions (or constraints) on its shape, e.g., monotone, concave, convex, etc. One typical example can be monotone dose-response curves. A Bayesian framework offers a probabilistic way of incorporating various shape constraints. A general approach is to expand the unknown function in a basis and translating the functional constraints to linear constraints in the coefficient space. This talk will revisit the problem of Bayesian shape-restricted inference in the light of a recently developed approximate Gaussian process that admits an equivalent formulation of the shape constraints in terms of linear restrictions on the basis coefficients. The focus will be on the proposed strategy to efficiently sample from the resulting constrained posterior by absorbing a smooth relaxation of the constraints in the likelihood and using circulant embedding techniques to sample from the unconstrained modified prior. This strategy also includes careful mitigation of the computational complexity arising from updating hyperparameters within the covariance kernel of the Gaussian process prior. The developed algorithm is shown to be accurate and highly efficient in simulated and real data examples.